By George Trujillo, Principal Data Strategist, DataStax
Increased operational efficiencies at airports. Instant reactions to fraudulent activities at banks. Improved recommendations for online transactions. Better patient care at hospitals. Investments in artificial intelligence are helping businesses to reduce costs, better serve customers, and gain competitive advantage in rapidly evolving markets. Titanium Intelligent Solutions, a global SaaS IoT organization, even saved one customer over 15% in energy costs across 50 distribution centers, thanks in large part to AI.
To succeed with real-time AI, data ecosystems need to excel at handling fast-moving streams of events, operational data, and machine learning models to leverage insights and automate decision-making. Here, I’ll focus on why these three elements and capabilities are fundamental building blocks of a data ecosystem that can support real-time AI.
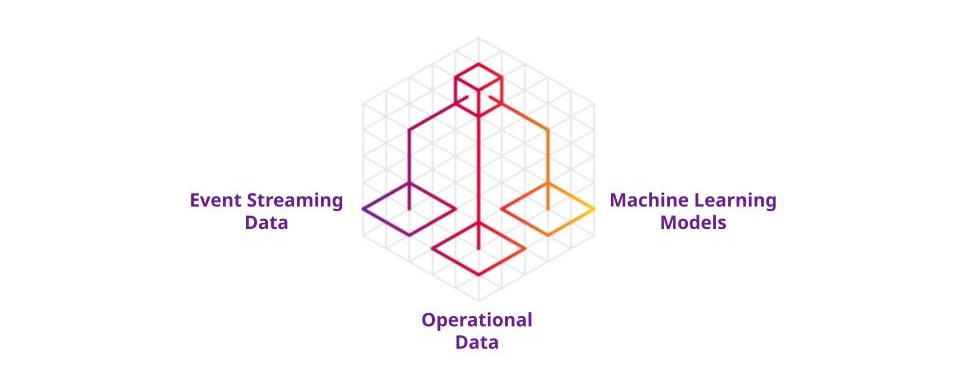
DataStax
Real-time data and decisioning
First, a few quick definitions. Real-time data involves a continuous flow of data in motion. It’s streaming data that’s collected, processed, and analyzed on a continuous basis. Streaming data technologies unlock the ability to capture insights and take instant action on data that’s flowing into your organization; they’re a building block for developing applications that can respond in real-time to user actions, security threats, or other events. AI is the perception, synthesis, and inference of information by machines, to accomplish tasks that historically have required human intelligence. Finally, machine learning is essentially the use and development of computer systems that learn and adapt without following explicit instructions; it uses models (algorithms) to identify patterns, learn from the data, and then make data-based decisions.
Real-time decisioning can occur in minutes, seconds, milliseconds, or microseconds, depending on the use case. With real-time AI, organizations aim to provide valuable insights during the moment of urgency; it’s about making instantaneous, business-driven decisions. What kinds of decisions are necessary to be made in real-time? Here are some examples:
Fraud It’s critical to identify bad actors using high-quality AI models and data
Product recommendations It’s important to stay competitive in today’s ever-expanding online ecosystem with excellent product recommendations and aggressive, responsive pricing against competitors. Ever wonder why an internet search for a product reveals similar prices across competitors, or why surge pricing occurs?
Supply chain With companies trying to stay lean with just-in-time practices, it’s important to understand real-time market conditions, delays in transportation, and raw supply delays, and adjust for them as the conditions are unfolding.
Demand for real-time AI is accelerating
Software applications enable businesses to fuel their processes and revolutionize the customer experience. Now, with the rise of AI, this power is becoming even more evident. AI technology can autonomously drive cars, fly aircraft, create personalized conversations, and transform the customer and business experience into a real-time affair. ChatGPT and Stable Diffusion are two popular examples of how AI is becoming increasingly mainstream.
With organizations looking for increasingly sophisticated ways to employ AI capabilities, data becomes the foundational energy source for such technology. There are plenty of examples of devices and applications that drive exponential growth with streaming data and real-time AI:
- Intelligent devices, sensors, and beacons are used by hospitals, airports, and buildings, or even worn by individuals. Devices like these are becoming ubiquitous and generate data 24/7. This has also accelerated the execution of edge computing solutions so compute and real-time decisioning can be closer to where the data is generated.
- AI continues to transform customer engagements and interactions with chatbots that use predictive analytics for real-time conversations.
- Augmented or virtual reality, gaming, and the combination of gamification with social media leverages AI for personalization and enhancing online dynamics.
- Cloud-native apps, microservices and mobile apps drive revenue with their real-time customer interactions.
It’s clear how these real-time data sources generate data streams that need new data and ML models for accurate decisions. Data quality is crucial for real-time actions because decisions often can’t be taken back. Determining whether to close a valve at a power plant, offer a coupon to 10 million customers, or send a medical alert has to be dependable and on-time. The need for real-time AI has never been more urgent or necessary.
Lessons not learned from the past
Organizations have over the past decade put a tremendous amount of energy and effort into becoming data driven but many still struggle to achieve the ROI from data that they’ve sought. A 2023 New Vantage Partners/Wavestone executive survey highlights how being data-driven is not getting any easier as many blue-chip companies still struggle to maximize ROI from their plunge into data and analytics and embrace a real data-driven culture:
- 19.3% report they have established a data culture
- 26.5% report they have a data-driven organization
- 39.7% report they are managing data as a business asset
- 47.4% report they are competing on data and analytics
Outdated mindsets, institutional thinking, disparate siloed ecosystems, applying old methods to new approaches, and a general lack of a holistic vision will continue to impact success and hamper real change.
Organizations have balanced competing needs to make more efficient data-driven decisions and to build the technical infrastructure to support that goal. While big data technologies like Hadoop were used to get large volumes of data into low-cost storage quickly, these efforts often lacked the appropriate data modeling, architecture, governance, and speed needed for real-time success.
This resulted in complex ETL (extract, transform, and load) processes and difficult-to-manage datasets. Many companies today struggle with legacy software applications and complex environments, which leads to difficulty in integrating new data elements or services. To truly become data- and AI-driven, organizations must invest in data and model governance, discovery, observability, and profiling while also recognizing the need for self-reflection on their progress towards these goals.
Achieving agility at scale with Kubernetes
As organizations move into the real-time AI era, there is a critical need for agility at scale. AI needs to be incorporated into their systems quickly and seamlessly to provide real-time responses and decisions that meet customer needs. This can only be achieved if the underlying data infrastructure is unified, robust, and efficient. A complex and siloed data ecosystem is a barrier to delivering on customer demands, as it prevents the speedy development of machine learning models with accurate, trustworthy data.
Kubernetes is a container orchestration system that automates the management, scaling, and deployment of microservices. It’s also used to deploy machine learning models, data streaming platforms, and databases. A cloud-native approach with Kubernetes and containers brings scalability and speed with increased reliability to data and AI the same way it does for microservices. Real-time needs a tool and an approach to support scaling requirements and adjustments; Kubernetes is that tool and cloud-native is the approach. Kubernetes can align a real-time AI execution strategy for microservices, data, and machine learning models, as it adds dynamic scaling to all of these things.
Kubernetes is a key tool to help do away with the siloed mindset. That’s not to say it’ll be easy. Kubernetes has its own complexities, and creating a unified approach across different teams and business units is even more difficult. However, a data execution strategy has to evolve for real-time AI to scale with speed. Kubernetes, containers, and a cloud-native approach will help. (Learn more about moving to cloud-native applications and data with Kubernetes in this blog post.)
Unifying your organization’s real-time data and AI strategies
Data, when gathered and analyzed properly, provides the inputs necessary for functional ML models. An ML model is an application created to find patterns and make decisions when accessing datasets. The application will contain ML mathematical algorithms. And, once ML models are trained and deployed, they help to more effectively guide decisions and actions that make the most of the data input. So it’s critical that organizations understand the importance of weaving together data and ML processes in order to make meaningful progress toward leveraging the power of data and AI in real-time. From architectures and databases to feature stores and feature engineering, a myriad of variables must work in sync for this to be accomplished.
ML models need to be built, trained, and then deployed in real-time. Flexible and easy-to-work-with data models are the oil that makes the engine for building models run smoothly. ML models require data for testing and developing the model and for inference when the ML models are put in production (ML inference is the process of an ML model making calculations or decisions on live data).
Data for ML is made up of individual variables called features. The features can be raw data that has been processed or analyzed or derived. ML model development is about finding the right features for the algorithms. The ML workflow for creating these features is referred to as feature engineering. The storage for these features is referred to as a feature store. Data and ML model development fundamentally depend on one another..
That’s why it is essential for leadership to build a clear vision of the impact of data-and-AI alignment—one that can be understood by executives, lines of business, and technical teams alike. Doing so sets up an organization for success, creating a unified vision that serves as a foundation for turning the promise of real-time AI into reality .
A real-time AI data ingestion platform and operational data store
Real-time data and supporting machine learning models are about data flows and machine-learning-process flows. Machine learning models require quality data for model development and for decisioning when the machine learning models are put in production. Real-time AI needs the following from a data ecosystem:
- A real-time data ingestion platform for messaging, publish/subscribe (“pub/sub” asynchronous messaging services), and event streaming
- A real-time operational data store for persisting data and ML model features
- An aligned data ingestion platform for data in motion and an operational data store working together to reduce the data complexity of ML model development
- Change data capture (CDC) that can send high-velocity database events back into the real-time data stream or in analytics platforms or other destinations.
- An enterprise data ecosystem architected to optimize data flowing in both directions.
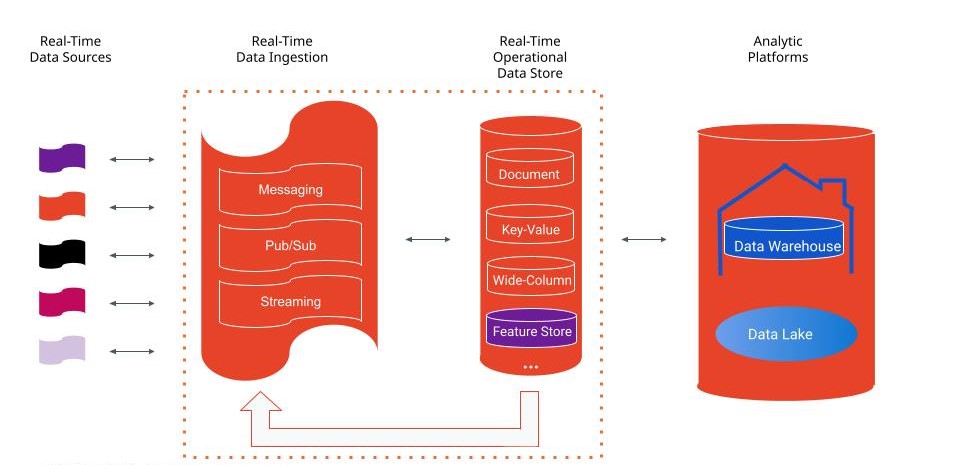
DataStax
Let’s start with the real-time operational data store, as this is the central data engine for building ML models. A modern real-time operational data store excels at integrating data from multiple sources for operational reporting, real-time data processing, and support for machine learning model development and inference from event streams. Working with the real-time data and the features in one centralized database environment accelerates machine learning model execution.
Data that takes multiple hops through databases, data warehouses, and transformations moves too slow for most real-time use cases. A modern real-time operational data store (Apache Cassandra® is a great example of a database used for real-time AI by the likes of Apple, Netflix, and FedEx) makes it easier to integrate data from real-time streams and CDC pipelines.
Apache Pulsar is an all-in-one messaging and streaming platform, designed as a cloud-native solution and a first class citizen of Kubernetes. DataStax Astra DB, my employer’s database-as-a-service built on Cassandra, runs natively in Kubernetes. Astra Streaming is a cloud-native managed real-time data ingestion platform that completes the ecosystem with Astra DB. These stateful data solutions bring alignment to applications, data, and AI.
The operational data store needs a real-time data ingestion platform with the same type of integration capabilities, one that can ingest and integrate data from streaming events. The streaming platform and data store will be constantly challenged with new and growing data streams and use cases, so they need to be scalable and work well together. This reduces the complexity for developers, data engineers, SREs, and data scientists to build and update data models and ML models.
A real-time AI ecosystem checklist
Despite all the effort that organizations put into being data-driven, the New Vantage Partners survey mentioned above highlights that organizations still struggle with data. Understanding the capabilities and characteristics for real-time AI is an important first step toward designing a data ecosystem that’s agile and scalable. Here is a set of criteria to start with:
- A holistic strategic vision for data and AI that unifies an organization
- A cloud-native approach designed for scale and speed across all components
- A data strategy to reduce complexity and breakdown silos
- A data ingestion platform and operational data store designed for real-time
- Flexibility and agility across on-premises, hybrid-cloud, and cloud environments
- Manageable unit costs for ecosystem growth
Wrapping up
Real-time AI is about making data actionable with speed and accuracy. Most organizations’ data ecosystems, processes and capabilities are not prepared to build and update ML models at the speed required by the business for real-time data. Applying a cloud-native approach to applications, data, and AI improves scalability, speed, reliability, and portability across deployments. Every machine learning model is underpinned by data.
A powerful datastore, along with enterprise streaming capabilities turns a traditional ML workflow (train, validate, predict, re-train …) into one that is real-time and dynamic, where the model augments and tunes itself on the fly with the latest real-time data.
Success requires defining a vision and execution strategy that delivers speed and scale across developers, data engineers, SREs, DBAs, and data scientists. It takes a new mindset and an understanding that all the data and ML components in a real-time data ecosystem have to work together for success.
Special thanks to Eric Hale at DataStax, Robert Chong at Employers Group, and Steven Jones of VMWare for their contributions to this article.
Learn how DataStax enables real-time AI.
About George Trujillo:
George is principal data strategist at DataStax. Previously, he built high-performance teams for data-value driven initiatives at organizations including Charles Schwab, Overstock, and VMware. George works with CDOs and data executives on the continual evolution of real-time data strategies for their enterprise data ecosystem.
Artificial Intelligence, IT Leadership
Read More from This Article: Unlocking the Power of AI with a Real-Time Data Strategy
Source: News