The combination of AI and search enables new levels of enterprise intelligence, with technologies such as natural language processing (NLP), machine learning (ML)-based relevancy, vector/semantic search, and large language models (LLMs) helping organizations finally unlock the value of unanalyzed data.
Search and knowledge discovery technology is required for organizations to uncover, analyze, and utilize key data. However, a deluge of data means legacy search systems can struggle to help business users quickly find what they need. In response, modern search systems have made great leaps in the accuracy, relevancy, and usefulness of results by leveraging AI-based capabilities. Now, a new wave of AI — generative AI (GenAI) — is changing how forward-looking organizations approach search, knowledge management, and other forms of knowledge discovery.
Search is proving foundational to connect GenAI with business and real-world data by retrieving pertinent information from enterprise data sources, a process called retrieval augmented generation (RAG). By augmenting GenAI models with related information, search systems work to ensure accurate, relevant, useful answers and insights. Real-world questions and actionable insights are where the rubber of AI meets the road of real business context, and the importance of the retrieval step in RAG cannot be understated.
Before diving into RAG, let’s take a step back to understand how we got here. This is helpful for understanding where best to utilize different forms of AI and information retrieval to get the most out of technology investments and accelerate the path toward becoming an AI-first organization.
How did we get here?
Over the past 10+ years, IDC has periodically surveyed organizations about the challenges and benefits of enterprise search and knowledge discovery. Survey questions focus on some of the untapped value represented by “hidden” or unanalyzed data. We poll knowledge workers on how much time they lose on a weekly basis to search-related activities like looking for information that they never actually find, searching across multiple data sources for a single piece of information, or connecting the dots between multiple pieces of information to arrive at an insight or answer.
In aggregate across 2013, 2015, 2019, and 2023, the data from these questions shows that legacy search engines that have not significantly advanced in the last five years have struggled to keep up with the increasing volume and variety of organizational data. These legacy engines typically use traditional keyword search and brittle, rules-based systems instead of adaptive, intelligent, semantic, and hybrid search. As a result, organizations using these tools must cope with poor relevancy ranking, outdated or broken query understanding, and basic findability challenges.
On the other hand, the research shows that search systems that kept up with AI innovations have advanced markedly in the past five years. AI has brought significantly better capabilities for searching, translating, and combining information. These capabilities include:
- Increasingly sophisticated natural language understanding, allowing more users to ask questions in more natural language
- ML-based relevancy ranking, improving the order in which results are displayed and enabling personalization as well as popularity-based reranking
- Semantic/vector search, further enhancing natural language search capabilities by expanding the semantic understanding of search systems beyond exact keyword matching. The combination of keyword and vector search (a.k.a. hybrid search) is especially popular for ecommerce search use cases due to the ability to find both exact SKUs/product names as well as recommended or similar products, improving conversion, cross-sell, and upsell
Using modern search meant that employees spent 12 fewer hours a week in time lost to search-related activities in 2023 compared to 2019 — a significant productivity improvement.1 Meanwhile, customers are also more satisfied and more willing to spend. Retail organizations that adopted modern AI-powered search reported benefits such as increase in cost savings (39%), profits (35%), and customer satisfaction and engagement (34%), as well as the ability to direct resources to higher-value and/or revenue-generating tasks (25%).
The new imperative: From search to Search AI
Leaders across almost every industry face demands to leverage AI for business advantage and must accelerate their organization’s transition to becoming AI first. IDC found that 83% of IT leaders believe that GenAI models that leverage their own business’ data will give them a significant advantage over competitors.2 However, in January 2024, only 24% of organizations believed that their resources were extremely prepared for GenAI.3 The trials, errors, and successes of the past one to two years have shown that search technologies help to bridge the gap between GenAI and enterprise data via RAG. Compared to fine-tuning, which requires retraining an AI model, RAG can be a more cost-effective and less time-consuming method of supplementing LLMs with specific and/or proprietary data:
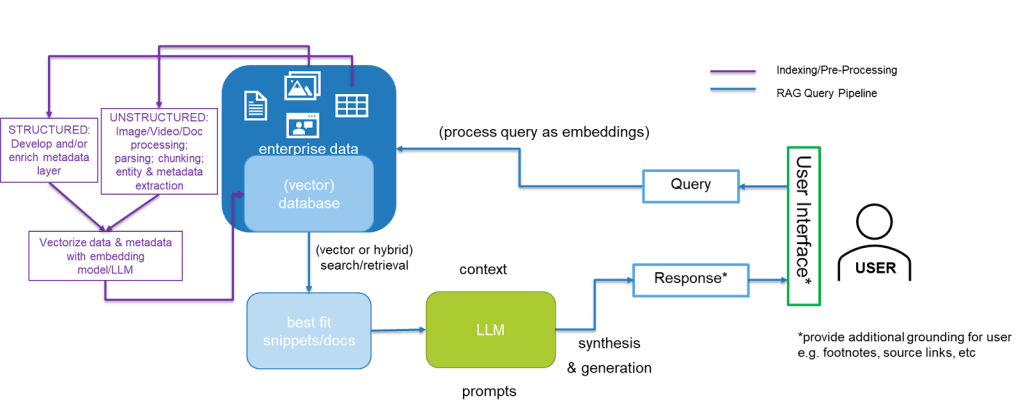
IDC
As the above diagram shows, there are a number of steps and technologies involved in RAG across the indexing/pre-processing and query pipelines. Depending on the type of data and the use case involved, either vector search or a combination of vector and keyword (a.k.a. hybrid) search may be needed. Companies should assess their resources to determine which of these pieces they want to build and maintain themselves. IDC recommends the following:
- Ensure the organization is leveraging strong search technology, with high levels of accuracy and relevancy, for the retrieval step of RAG. Aspects to look for include hybrid search (keyword and semantic/vector), automated reranking, and low-code/no-code tools that make testing and tuning easy for a wide variety of users. This step is crucial for ensuring that LLMs provide the most relevant, useful, and actionable summaries and answers.
- Assess the accuracy and freshness of data sources, and consider what tools will be used to connect, filter, or ingest data into the pipeline. Ensure that data governance and business rules, such as access permissions, are not lost in the process and that the system has strong security guardrails.
- Determine what types of AI are best suited to different use cases. GenAI should be applied strategically to ensure that its usage is feasible, valuable, and responsible. If needed, select a provider with the necessary experience to assist in prioritizing use cases and AI usage.
- Look for a partner that can assist with some or all of the steps required to connect enterprise data to LLMs, including parsing, chunking, embedding, storing, and using vector or hybrid search to retrieve key information to feed to the LLM.
See how AI is changing the search game — plus get essential guidance for building modern search with your proprietary data. Get the IDC Infobrief.
Hayley Sutherland is a Research Manager for Conversational AI and Intelligent Knowledge Discovery within IDC’s Software market research and advisory group. Her core research coverage includes conversational AI and search, with a focus in AI software development tools and techniques for chatbots and digital assistants, speech AI and text AI, machine translation, embedded knowledge graph creation, intelligent knowledge discovery, and affective computing (also known as emotion AI).
1IDC’s North America Knowledge Discovery Survey, February 2023, n = 522
2IDC’s GenAI ARC Survey, August 2023, n=1,363
3IDC’s Future Enterprise Resiliency and Spending Survey, Wave 1, January 2024, n = 881
Read More from This Article: How search accelerates your path to “AI first”
Source: News