The road ahead for IT leaders in turning the promise of generative AI into business value remains steep and daunting, but the key components of the gen AI roadmap — data, platform, and skills — are evolving and becoming better defined.
That was the key takeaway from the “What’s Next for GenAI in Business” panel at last week’s Big.AI@MIT event, moderated by Lan Guan, CAIO at Accenture.
Guan, along with AI leaders from S&P Global and Corning, discussed the gargantuan challenges involved in moving gen AI models from proof of concept to production, as well as the foundation needed to make gen AI models truly valuable for the business.
It’s a position many CIOs find themselves in, as Guan noted that, according to an Accenture survey, fewer than 10% of enterprises have gen AI models in production.
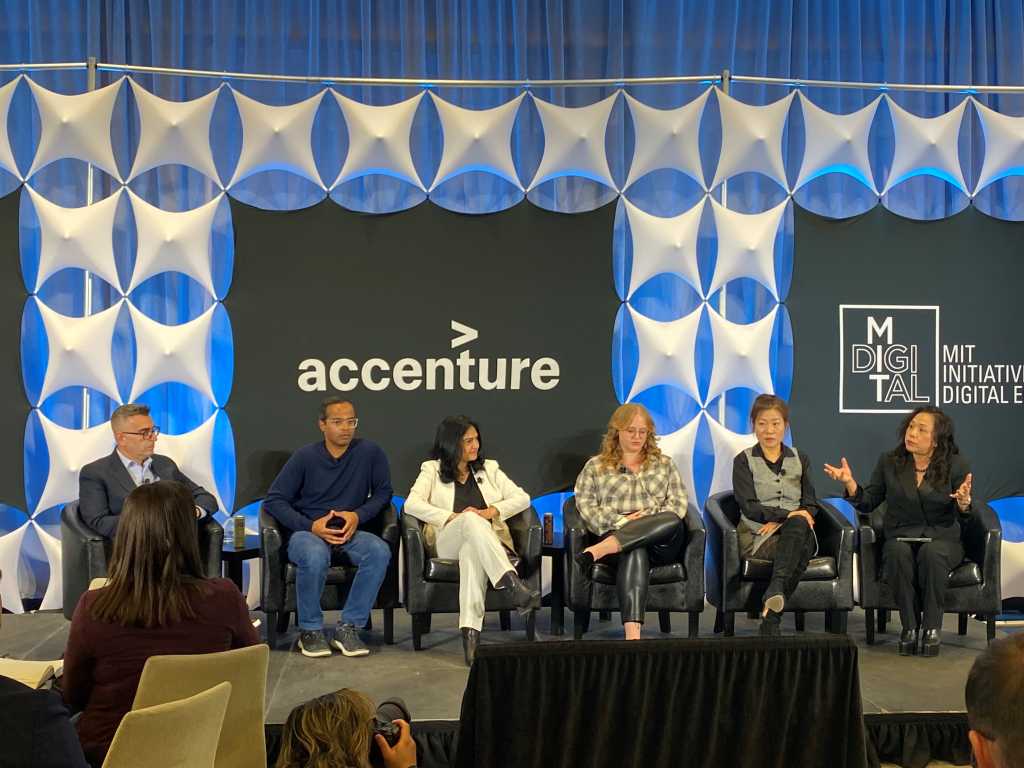
“What’s Next for GenAI in Business” panel at last week’s Big.AI@MITevent, moderated by Lan Guan, CAIO at Accenture
Accenture
“98% of business leaders say they want to adopt AI, right, but a lot of them just don’t know how to do it,” claimed Guan, who is currently working with a large airliner in Saudi Arabia, a large pharmaceutical company, and a high-tech company to implement generative AI blueprints in-house.
Unfortunately, despite hard-earned lessons around what works and what doesn’t, pressure-tested reference architectures for gen AI — what IT executives want most — remain few and far between, she said.
Progress — and challenges
Accenture’s work with Saudia Airlines involves a “travel companion” model that is far more than an online travel agency, reservation agent, or travel guide, Guan said. The airliner, which competes against Qatar Airlines, is counting on agentic AI and the LLM to elevate its bookings and expand its share of the growing market, she said, adding that the six-month-old model has attracted 3 million visitors and has handled some bookings, but its value is far more strategic.
“Their main intent is to change perception of the brand. Give a better experience,” she said. “I cannot say I have abundant examples like this.”
Soumya Seetharam, CDIO at Corning, said the manufacturer has been on its data journey for a few years, with more than 70% of its business transaction data being ingested into a data platform. But that’s only structured data, she emphasized.
“Our big challenge, honestly, is the unstructured data,” Seetharam said, noting that Corning must now “figure out how to categorize [unstructured data] and bring it in a form that can be useful.”
Bhavesh Dayalji, CAIO at S&P Global, added that integrating all kinds of data structures into gen AI models is a challenge. To that end, the financial information and analytics firm is developing APIs and examining all methods for “connecting your data to large memory models.”
S&P Global has also created evaluation sets to test not only gen AI models for hallucinations but to ensure there is a common method of evaluating outcomes and efficacy. As experts in financial services and commodity markets, there must be standard evaluation methods, he said.
“Then we need to bring that to the [financial services] community as a whole for evaluating [generative AI] models and solutions,” said Dayalji, who is also CEO of Kensho, S&P Global’s AI innovation hub. “I think driving down the data, we can come up with some kind of solution.”
A roadmap emerges
Accenture’s AI chief offered three key pieces of advice for CIOs to want to maximize gen AI ROI — without succumbing to “garbage in, garbage out” failures.
First and foremost, clean up all corporate data and prepare it properly for use in foundational gen AI models, Guan told CIO.com during a private interview after the panel.
“They are not cleaning up their data,” she said, warning that although many CIOs are taking up the data challenge most aren’t preparing data properly for gen AI. “Proprietary data is your biggest competitive advantage.”
Second, Guan said, CIOs must take a “platforms-based approach” to AI development and deployment. Enterprises can run gen AI workloads on the mainframe, for example, but most of the activity will run on the public cloud or on-premises private clouds, she said.
“It’s time for them to actually relook at their existing enterprise architecture for data and AI,” Guan said.
“A platform-based approach to AI emphasizes building a scalable, reusable foundation that evolves with the organization, rather than developing costly, siloed solutions for individual use cases,” said Guan, supporting the notion that establishing standards to test outcomes of models is necessary.
“By identifying commonalities across use cases — such as data pipelines, model management, and applications — the organization can create shared components that streamline deployment, reduce redundancy, and accelerate time-to-value for AI solutions and enterprise reinvention.”
Accenture has developed its own AI Refinery — a platforms-based approach to gen AI model creation and deployment.
“In practical terms, the AI Refinery is a cognitive architecture, with built-in intelligence and context, where AI agents can reason, plan, and work alongside humans to dynamically execute tasks,” Guan said. “Using LLMs, the system interprets human intentions and adapts based on experience, integrating organizational knowledge for better decision-making.”
Finally, CIOs must ensure they have a good stable of qualified AI personnel and a lineup of specialized skills for building and deploying gen AI models, she advised. Most CIOs are not upskilling or training employees fast enough, and the availability of a wide range of talents is thin, including data scientists and software engineers with GPU infrastructure planning skills.
Guan herself was a data scientist at Accenture six years ago and started working on software engineering skills, application development skills, and infrastructure planning skills to prepare for gen AI. Finding talent is “a challenge that I am also facing,” Guan said.
At the summit, a wide range of AI startups and solution providers were in attendance showcasing solutions that claim to solve various AI challenges facing CIOs and CAOs. On a separate panel, venture capitalists from New Technology Ventures and Underscore VC said the investment dollars for innovation are in abundance but acknowledge the market is still in a hype cycle.
Yet, as the moderator of the VC panel pointed out, most players know generative AI is a transformative technology.
“People recognize how tectonic this shift is … and people are jumping in,” said Heidi Messer, a VC and co-founder of gen AI startup Collective[i], which offers a financial forecasting service. “We do have to read through the noise and it gets tricky, but over the next five to seven years there’s going to be [major] value created.”
In the meanimte, S&P’s Dayalji said enterprises must think beyond specialized AI skills to envision all aspects of the new roles that will be created as a result of employee interaction with gen AI models and AI agents handling mundane tasks.
“We’re continuing to educate our workforce and making sure they understand the impact of AI on their roles and how they do their roles,” he said. “Secondly, how do you give them tools to do different work and innovate?”
Read More from This Article: CIOs contend with gen AI growing pains
Source: News