By Chad Smykay
Companies have access to more data than ever before – but it doesn’t mean everyone in an organization trusts the reliability of that data and resulting analytics. A company may be filled with data engineers and analysts with tremendous individual virtuosity when it comes to dissecting and utilizing data, but that doesn’t necessarily create organization-wide trust of data.
Companies need a way to manage their data that systematically and consistently ensures the analytics come from reliable datasets that everyone can have faith in. The only way to do this is to build in trust as part of a company’s data architecture. Such an architecture also means the data produces better, more actionable answers.
A recent Gartner® report, Predicts 2022: Data and Analytics Strategies Build Trust and Accelerate Decision Making, discusses the key elements important for building trust and accelerating decision making. According to Gartner, Inc., “As Figure 1 shows, D&A leaders and their current D&A strategies need to support and scale measurable business impact by applying trusted D&A to the organizations decision-making capabilities.” ¹
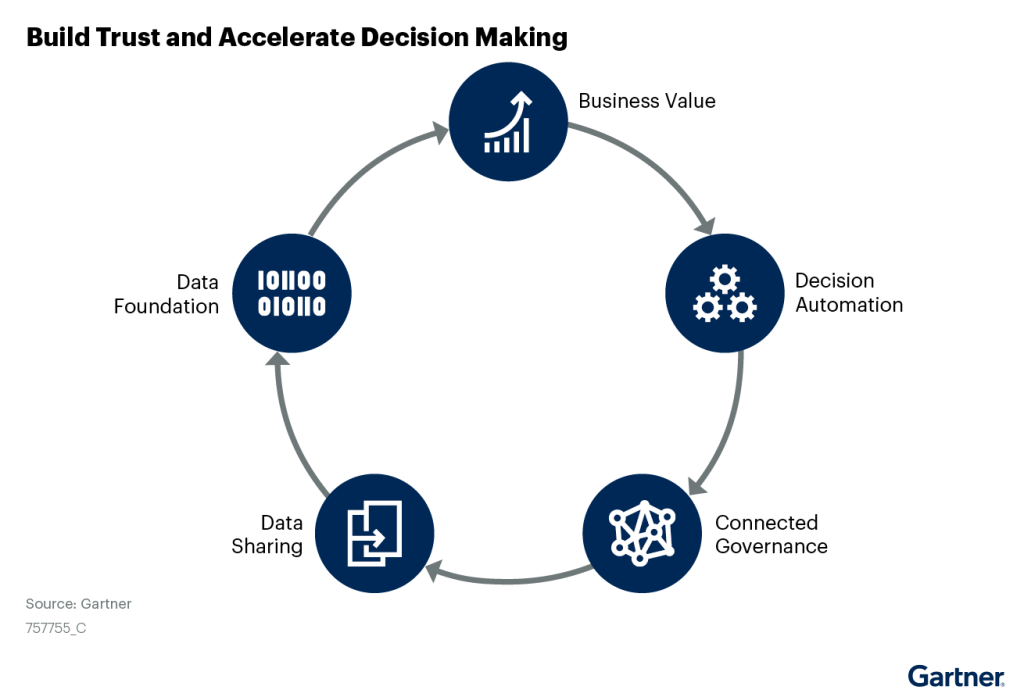
Figure 1. Build Trust and Accelerate Decision Making
Through years of working with enterprises across a variety of industries, it’s become clear that using a robust data fabric, which ensures all data is integrated into a single place, is critical to building trust across these areas. That data fabric does not necessarily have to extend across the entire business — individual departments or divisions can implement their own data fabrics. While these individual data fabrics may foster data silos, they also ensure data trust within segments of the business, allowing for collaboration on larger, more substantial problems. This trust and collaboration are vital because these problems cannot be undertaken if companies do not have the right processes and architectures in place first.
Read on to learn how data fabrics can help organizations make significant strides in these key areas so they can deliver business value and get ahead of the competition.
Decision automation
In today’s enterprises, data can and should be used to automate processes, including decision-making. For instance, a bank can use automated decision-making to churn through customers’ credit and financial history and decide who is worthy of receiving a loan. But for automation to be successful and for users to trust the results, companies must understand how the automated systems arrive at decisions based on the available data.
The right approach to automation is a layered one. First, set up the tools correctly that will use the data, then establish strong data pipelines to process the data. From there, analytics can be automated to analyze the data, which produces insights and answers for human users more quickly. If this occurs, then the automation leads to standardized and predictable answers based on standardized data. This builds trust because users know the automated processes are producing reliable results based on solid data sources.
Connected governance
Ultimately, governance is a people, process, and technology problem. The goal of governance is to ensure a company knows what every user is doing with the available corporate resources (whether that is data or staff) at any given time.
In a disconnected governance structure, data usage is a black box. In this model, a company has no idea at any given time who is using specific resources, or what they are using those resources for. This leaves companies vulnerable in terms of security, privacy, and compliance. It can also create significant inefficiencies, where multiple analysts are working on the same problem and creating duplicate datasets in the process.
Connected governance, comparatively, is the opposite: a white or clear box. In this model, companies have full transparency about who is using specific datasets and why they’re using those datasets. With connected governance, the whole story of how resources are being used is available to everybody participating on a particular project. Over time, connected governance can incorporate zero trust principles, so users or applications can only access the data they have been authorized to use.
Connected governance increases trust because users know the data they have access to and are confident they can use it in the ways they need. They also gain trust in the datasets themselves, as they can see who worked on every data source and how it has been used up to that point.
Data sharing
With the high turnover of data engineers in many organizations, companies must avoid data architectures that limit data sharing, as projects should not die just because an individual analyst or team leaves the business. Proper data sharing means insights are shared among individuals and across the organization in a consistent and ongoing manner. This helps businesses push forward discoveries and allows users to continue working on projects that have been started in the past.
There are multiple ways to achieve this type of data sharing, from automated to manual processes. Data fabrics help companies support data sharing for multiple, different types of data, whether it’s located in a database, a table, a file, or a real-time event stream. The data fabric architecture does this, as noted earlier, by ensuring all data is integrated into a single place. The result: it becomes easier to share results, capture insights, and then make decisions based on what has been learned. This can be done through the development of models that users come to trust and which can be shared and reused over time.
Data foundation
A strong data foundation provides a data infrastructure that ensures a standardized process for how data is acquired, absorbed, created, backed up, managed, cleaned, and used across its lifecycle. This foundation combines the data management and data lifecycle processes with the actual hardware and software that support them. A data fabric is compatible with almost any tool used to establish this data foundation.
The more solid a company’s data foundation, the better and more reliable the organization’s data will be. Data becomes more transparent, from its lineage to its usage, which means it is readily apparent when something goes wrong. This builds trust because users have an efficient and reliable data system that allows them to increase the velocity at which they can leverage data to make decisions.
Establishing trust to deliver business value
Establishing organizational-wide trust of data with a data fabric is not something that can be accomplished overnight – especially for large organizations. Companies should go step-by-step over time, keeping a focus on business value, and build on each incremental success to establish the foundational architecture and processes that make true trust possible. As with any relationship, trust grows over time.
To read more about how data fabrics solve common data science and analytics challenges for large data-driven enterprises, check out, How to Simplify Your Approach to Data Analytics
Gartner, Predicts 2022: Data and Analytics Strategies Build Trust and Accelerate Decision Making, Jorgen Heizenberg et.al, 2 December 2021
GARTNER is a registered trademark and service mark of Gartner, Inc. and/or its affiliates in the U.S. and internationally and is used herein with permission. All rights reserved.
¹Predicts 2022: Data and Analytics Strategies Build Trust and Accelerate Decision Making.
Published 2 December 2021, By Jorgen Heizenberg, Lydia Clougherty Jones, Ted Friedman, Andrew White, Saul Judah, Gareth Herschel, Rita Sallam, Ehtisham Zaidi, Svetlana Sicular
____________________________________
About Chad Smykay
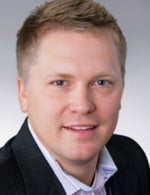
Chad Smykay, Field CTO, HPE Ezmeral Data Fabric, has extensive background in operations with his time at USAA as well as helping to build many shared services solutions at Rackspace, a world class support organization. He has helped implement many production big data/data lake solutions. As an earlier adopter of Kubernetes in the application space coupled with data analytics use cases, he brings a breadth of background in the application modernization space for business use cases.
Read More from This Article: How Data Fabrics Build Trust for Data and Analytics Success
Source: News