Have you ever gazed upon a Monet painting and lost yourself for a time? I have. I love great works of art. The University of London’s research says beautiful art catalyzes an instant release of dopamine into the brain. I feel that jolt of reward and motivation when I see a masterpiece.
As an artist and an engineer, I find myself curious about the techniques, stories of the paintings, reasons behind the color choices, the style of brushstrokes, and the preservation of each piece. I started studying art to give myself a break from the high-tech world. Now, ironically, the art world is being disrupted by emerging technology–specifically generative AI tools such as OpenAI’s ChatGPT, Google’s Bard, and Meta’s LLaMa. These tools enable me to discover the information I am seeking—and do so in near real-time and in novel, profound ways.
Art, business, and generative AI
Art has existed since the dawn of humankind, giving us a window into history and stories waiting to be revealed. Each masterpiece is more than just the marks on canvas; it’s the culmination of a culture, an artist, and influence. Art is an ideal example of how generative AI can create a massive amount of value by extending the way we look at artwork.
Generative AI is a way to create augmented information that expands our understanding of an image and the ephemera around it. For instance, if you start with a Monet painting and use an extended generative AI model trained on all Monet artwork and complementary artists, you can create an input dataset that includes images by the artist. The dataset is complemented by a text-based dataset sourced from the entire internet. You can ask generative AI to tell you about the Monet image, and it will generate an essay about Oscar-Claude Monet and that specific painting. Then, you can use the essay to create more information with direct and indirect correlations, such as to another oil painting, related modern impressionist art, or a different Monet image. Generative AI does this based on a knowledge graph, a network of related concepts.
Simply put, generative AI is a powerful way to exponentially expand a small amount of information into a very large understanding, which in turn leads to smarter, more informed decisions.
Powerful potential but less than perfect
Generative AI is rapidly moving into the business world, across industries and companies of all types and sizes. Companies such as Salesforce, Amazon, The Coca-Cola Company, and Snapchat are making bold moves to integrate generative AI into a host of capabilities. Generative AI has the potential to revolutionize many aspects of our lives, but ethical considerations must be addressed when developing and deploying generative AI models.
Ethical considerations must be a fundamental part of the development and deployment of generative AI models to ensure they are used in ways that are fair, safe, and beneficial for society as a whole. For that, generative AI needs explainability.
4 ways to enable explainability in generative AI
Creating explainability in a generative AI model can help build trust in the models and the confidence to develop enterprise-level use cases. Explainability requires careful consideration and planning throughout the entire development process. (You can even ask ChatGPT about this.)
Here are some key guidelines:
- Model simplification. As AI models become more complex, understanding how they arrive at decisions or predictions becomes more difficult. Deep learning models, for example, can have thousands or even millions of parameters. Model architecture can be simplified by reducing the number of layers in the neural network, which enables understanding and improves interpretation of the model’s decisions.
- Interpretability tools. Several tools are available that can help developers interpret the decisions made by a generative AI model. For example, model trainers can use attention maps to visualize which parts of an input image are most important for the output. Model developers can use other tools, such as decision trees or feature importance plots, to identify the key factors that influence a model’s decision.
- Referenceable data. Training data must be referenceable and audited for quality control. This helps flag unintentional bias, prevent unfair decisions, and enable ongoing improvements, including provenance for traceability.
- Human oversight. In some cases, you may need a human in the loop to provide oversight and ensure that the generative AI model is making decisions in a responsible and ethical manner. For example, a human could be tasked with reviewing the output of a model to ensure it’s not generating harmful or biased content. Using diverse groups of people to access data sets and help identify non-obvious biases has proven to be an effective method of detecting unintentional heterogeneity, which leads to model bias. In addition, trained people from different backgrounds can review algorithms using company or government policies and standards to look for potential ethical challenges with the use of data or in the outputs of the models.
Moving ahead
Generative AI has the potential to transform many aspects of our lives, including the world of art. We already use models to create new works of art, preserve existing works, and expand our understanding of art history. For example, researchers have used AI to restore a painting by Rembrandt that was damaged by fire. The AI model analyzed other works by Rembrandt to generate a new image that closely matched the original painting.
As generative AI evolves and extends value into more enterprise use cases, IT leaders, technologists, and developers must adopt a holistic approach that considers the technical, ethical, and social aspects of AI explainability and involves all relevant stakeholders in the development and deployment of AI models. By doing so, we can ensure that we use generative AI in ways that benefit society as a whole.
How we collectively work toward responsible use of generative AI is the story we want future generations to discover when they see the masterpieces we build with this powerful capability.
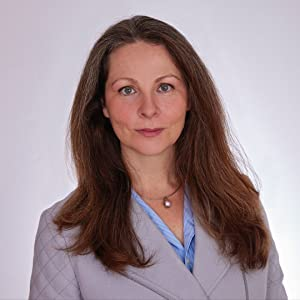
Nicole Reineke is senior vice president of innovation at Iron Mountain
Iron Mountain
Nicole Reineke is senior vice president of innovation at Iron Mountain. Prior to this role, she was a senior distinguished engineer in the office of the CTO at Dell Technologies. Over the last 20 years, she has founded and led high-tech companies in product executive leadership roles establishing expertise in areas such as sustainability-aware infrastructure, data trust, blockchain, hybrid cloud, artificial intelligence/machine learning, artificial intelligence ethics, augmented and virtual reality, data center management, and intelligent data management. She has 14 patents, awaits grants on 75 additional filings, and is the co-author of “Compassion-Driven Innovation: 12 Steps for Breakthrough Success.” She is a passionate hobbyist—a pianist, dancer, and artist—who enjoys hiking with her dog and family.
Fraud Protection and Detection Software
Read More from This Article: 4 ways to enable explainability in generative AI
Source: News